Introduction
Seismic data interpretation is a cornerstone in fields such as oil and gas exploration, environmental studies, and geotechnical engineering. At the heart of this interpretation process lies velocity model building, a technique that maps seismic wave velocities within the Earth’s subsurface. Traditionally, this process demanded labor-intensive manual analysis and computations, often limited by human expertise and time constraints. However, with the advent of machine learning, velocity model building has seen transformative improvements in efficiency, accuracy, and scalability. This article delves into how machine learning is reshaping the process of velocity model building from raw shot gathers, providing a comprehensive overview of its applications, benefits, and methodologies.
Understanding Raw Shot Gathers
velocity model building from raw shot gathers using machine learningorm the foundation of seismic data processing. Each shot gather consists of seismic data recorded at various geophones or receivers following a single seismic event, known as a “shot.” These gathers contain crucial information about subsurface structures, but they are inherently noisy and complex.
In the past, preprocessing raw shot gathers to extract meaningful insights required expert intervention. Today, machine learning algorithms can automate much of this process, reducing errors and improving data quality. By efficiently handling noise and inconsistencies, machine learning helps geophysicists focus on deeper analysis rather than data cleanup.
Importance of Velocity Model Building From Raw Shot Gathers Using Machine Learning
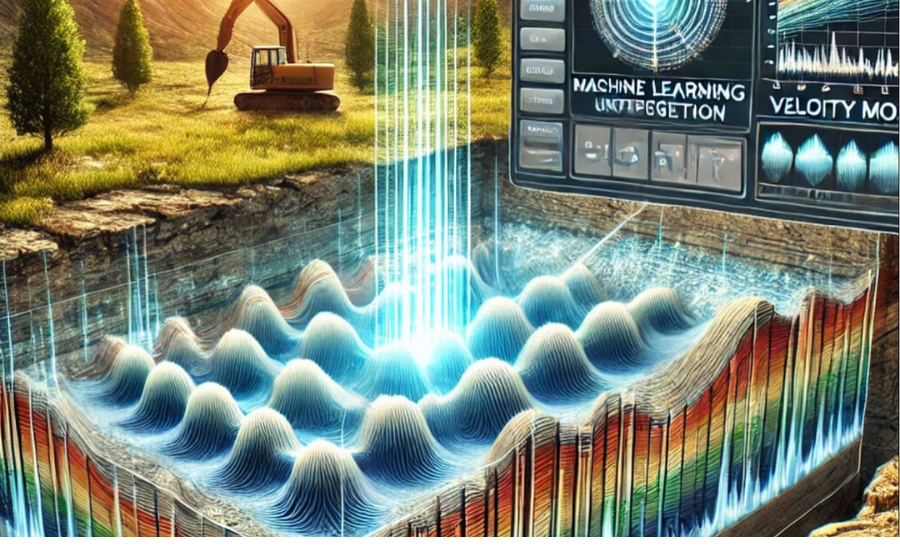
Velocity models are essential tools in seismic interpretation, as they describe how seismic waves travel through various subsurface layers, offering valuable insights into rock types, fluid distributions, and geological formations. Accurate Velocity model building from raw shot gathers using machine learning allows geophysicists to produce detailed seismic images, which play a crucial role in locating natural resources such as oil, gas, and minerals. They also help assess geological risks, including fault lines and earthquake zones, and optimize drilling operations to prevent costly mistakes. On the other hand, inaccuracies invelocity model building from raw shot gathers using machine learning can lead to erroneous interpretations, resulting in financial losses or missed opportunities. Therefore, enhancing the accuracy and efficiency of velocity model building is a top priority for the industry.
Traditional Methods Of Velocity Model Building
They interpreted shot gathers through trial-and-error methods, aiming to refine the models over time. This process involved manually identifying key subsurface features, simulating wave propagation to validate the models, and making iterative adjustments to enhance accuracy. While this method was effective, it had several significant limitations. It was extremely time-consuming, often taking weeks or months to develop a single velocity model. Additionally, manual interpretation introduced subjectivity and inconsistencies, making the process prone to human error. Furthermore, traditional methods struggled to handle the increasing size and complexity of modern seismic datasets, highlighting the need for more efficient approaches.
Challenges In Velocity Model Building
Building Velocity model building from raw shot gathers using machine learning presents several significant challenges. Raw shot gathers are often noisy, with interference stemming from environmental factors, equipment errors, and surface waves, making data preprocessing a critical step. Additionally, the inversion process used to derive velocity model building from raw shot gathers using machine learning is inherently ill-posed, meaning that even small variations in the data can lead to substantial discrepancies in the final model. Subjectivity is another issue, as different interpreters may produce varying results from the same dataset, leading to inconsistencies. Moreover, the process of simulating wave propagation to refine velocity model building from raw shot gathers using machine learning is computationally intensive, requiring substantial resources. These challenges highlight the need for automation and advanced computational techniques, positioning machine learning as a transformative tool in velocity model building
Machine Learning In Seismic Data
Machine learning (ML) offers a powerful solution to the challenges of velocity model building by providing a robust framework for seismic data analysis. Unlike traditional methods, ML algorithms can analyze large datasets to uncover patterns and relationships that might be overlooked through manual interpretation. One of the key benefits of ML is its ability to automate tasks, significantly reducing the dependence on human interpreters and minimizing subjectivity. Additionally, ML accelerates the data processing and model generation phases, enabling faster results. Its scalability is another major advantage, as it can efficiently handle the increasingly large datasets generated by.
From Raw Shot Gathers To Velocity Model Building From Raw Shot Gathers Using Machine Learning: Process Overview
The first step is data preprocessing, which involves removing noise and correcting distortions caused by surface and near-surface layers of the seismic data. Next, feature engineering is performed, where meaningful attributes such as travel time, amplitude, and frequency content are extracted from the shot gathers. Following this, model training takes place, where machine learning models are trained on labeled shot gather data to predict the velocity model building from raw shot gathers using machine learning. Finally, the predictions are validated by comparing them with results from traditional methods or additional data to ensure their accuracy.
Data Preprocessing for Machine Learning
Data preprocessing is a critical step in preparing velocity model building from raw shot gathers using machine learning or machine learning. This process involves several key techniques to ensure the data is ready for model training. First, noise reduction is applied to filter out unwanted signals, often using methods like band-pass filtering, to remove irrelevant frequencies and reduce environmental noise. Finally, data augmentation techniques are used, where synthetic data is generated to expand the dataset, helping improve the robustness and generalization of the machine learning model during training.
Feature Engineering from Shot Gathers
Feature engineering involves extracting relevant attributes from seismic data that are crucial for building accurate velocity model building from raw shot gathers using machine learning. Key features commonly derived from shot gathers include travel time, which measures the duration it takes for seismic waves to reach various receivers; amplitude, which indicates the strength of the seismic signal and provides insights into subsurface properties; and frequency content, which helps differentiate between geological layers. Additionally, dimensionality reduction techniques, like Principal Component Analysis (PCA), are frequently applied to simplify the data without losing essential information, ensuring that the machine learning models can operate efficiently on complex seismic datasets.
FAQs About Velocity Model Building From Raw Shot Gathers
Q. Why are velocity model building from raw shot gathers using machine learning important in seismic data interpretation?
A: Velocity models provide crucial insights into subsurface structures, aiding in resource exploration, risk assessment, and drilling optimization.
Q. What challenges do traditional velocity model building methods face?
A: Traditional methods are time-consuming, prone to human error, and struggle with large datasets.
Q. How does machine learning improve velocity model building?
A: Machine learning automates data interpretation, handles large datasets efficiently, and improves the accuracy of velocity model building from raw shot gathers using machine learning.
Q. What types of machine learning are used in seismic data processing?
A: Supervised, unsupervised, and reinforcement learning are commonly used in seismic applications.
Q. What is feature engineering in seismic data processing?
A: Feature engineering involves extracting relevant attributes from shot gathers, such as travel time and amplitude, to train machine learning models effectively.
Conclusion
Machine learning is revolutionizing velocity model building, transforming it from a labor-intensive process to a fast, accurate, and scalable solution. By automating critical tasks and improving the handling of complex datasets, ML enables geophysicists to generate high-quality velocity model building from raw shot gathers using machine learning with unprecedented efficiency. This not only enhances resource exploration and risk assessment but also opens new frontiers in seismic data interpretation.
Stay connected for exclusive updates and alerts! Chicago Daily